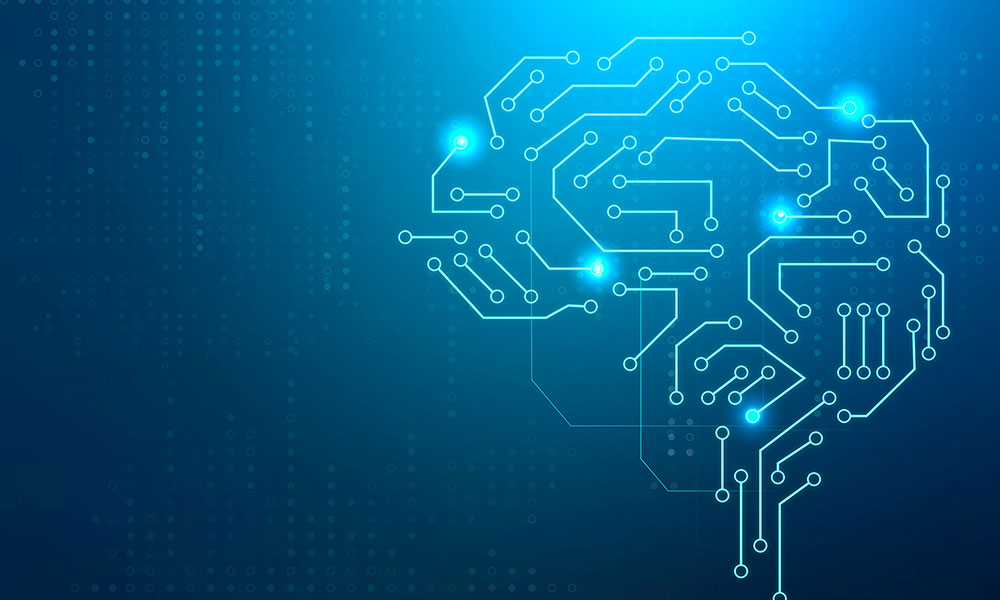
AI in Healthcare: Bridging the Gap Between Potential and Reality
When was the last time you went a full day without interacting with some kind of AI technology? Your last wilderness backpacking trip? The big blackout of 20-whatever-it-was? Never? The point is that today, we are surrounded by and immersed in AI—whether we’re browsing social media, shopping online, or using a maps app to navigate around a traffic jam.
But not in healthcare. The stark reality is that our industry lags far behind most others in AI adoption —in spite of its rich potential as a powerful predictive diagnostic tool.
So what explains this gap? Given the undeniable benefits AI can offer the healthcare industry, what’s holding us back? The (relatively) short answer revolves around some core challenges that are unique to our industry. And understanding what those challenges are—and how to address them—is an important first step in turning AI from a thought experiment into a practical, powerful tool for improving patient care.
Challenge #1: Taking the power of AI from the lab to the bedside
In our industry (more than most), there is a problematic gap—a kind of language barrier—between the data scientists who develop AI algorithms and the clinicians on the front lines of patient care.
For example, most data scientists simply don’t have the specific, in-depth healthcare knowledge to understand how to bring data from different systems and devices together in a clinical setting, access and use it without violating privacy regulations, and present mathematical insights to frontline providers in ways that add value and make sense. Today, these disconnects between mathematical algorithms and frontline care are clearly making it more difficult for healthcare organizations to fully embrace AI.
Challenge #2: Bringing data, AI models, and applications together
AI models require massive amounts of data to function. But in today’s healthcare world, it’s still far too difficult to access, combine, and transform raw, isolated data from different systems and devices into the usable fuel AI models need to generate meaningful insights. Clinicians also lack an effective delivery system—a care-focused application (or integration with an existing application)—that makes these new AI insights relevant, integrates them naturally into existing workflows, and frames them in a way that they value and understand.
Challenge #3: Building AI solutions that clinicians trust
In the current climate, many healthcare professionals are simply not convinced that today’s clinical AI algorithms are accurate, well-tested, or mature enough to meet their rigorous standards. To address this trust issue, data scientists and clinicians need a more transparent and collaborative process for designing, building, and testing clinical AI models—together with new tools, frameworks, and a common vocabulary they can use to bridge the gap between the language of algorithms and the language of care.
Finding the path forward
What will it take to bridge these gaps and overcome the challenges that are preventing the healthcare industry from fully embracing the full potential of AI? The answer starts with a flexible technology platform that lives in the space between data scientists and frontline clinicians—and focuses on providing the capabilities healthcare organizations need to address these core challenges and accelerate the use of AI in clinical settings.
Learn more about how this platform approach works and the challenges it addresses: