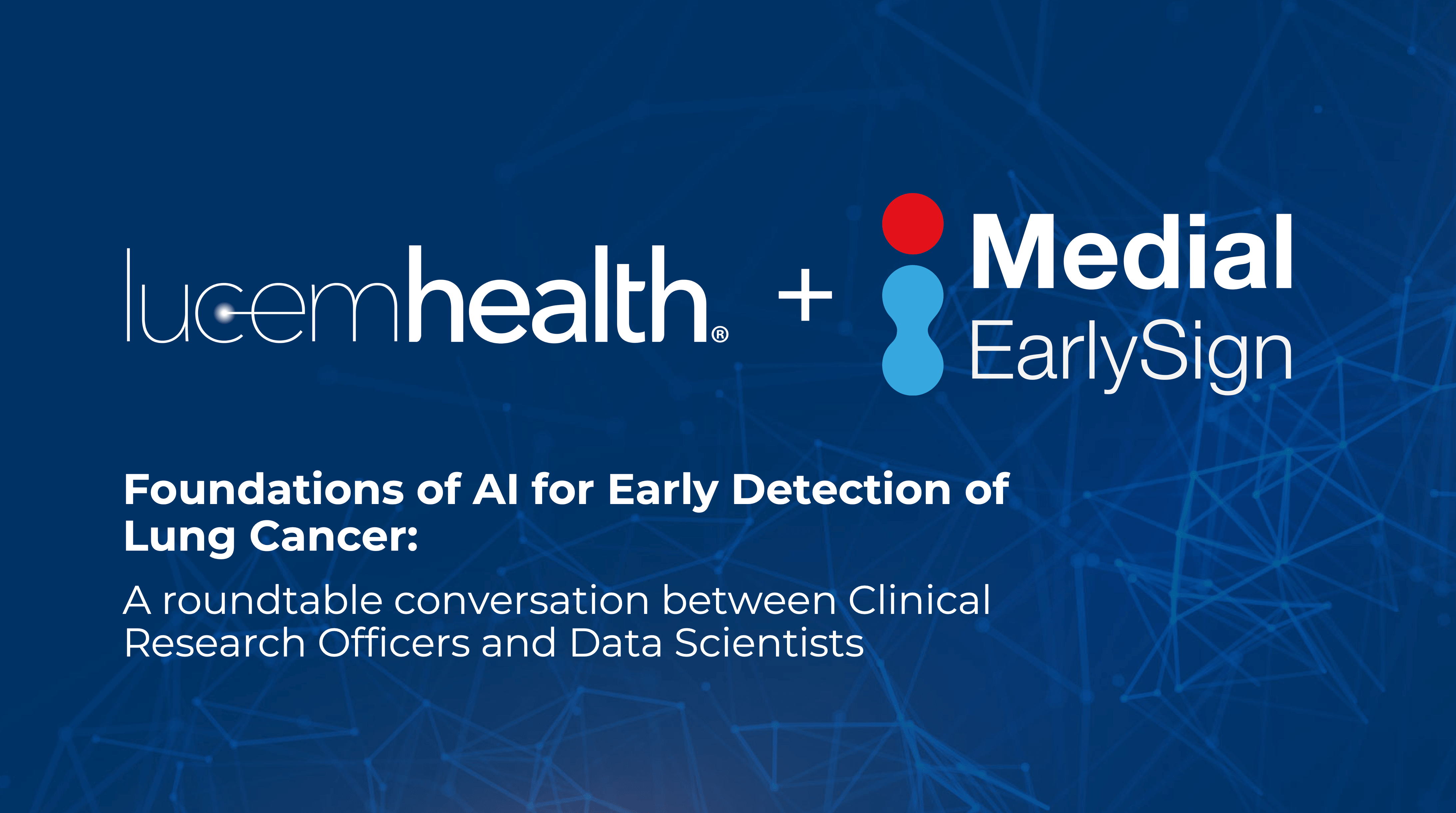
Foundations of AI for Early Detection of Lung Cancer: The Data Fueling AI Models
Introduction
We recently had the pleasure of hosting an expert data science roundtable called the “Foundations of AI for Early Detection of Lung Cancer,” which brought together domain experts including physicians, data scientists, and clinical researchers. These senior leaders from both Lucem Health and Medial EarlySign came together to discuss key aspects of model development for early detection of lung cancer:
- Optimal data strategies used to train models
- Exploration of the clinical, human, and financial impacts of early detection
- The importance of keeping physicians ‘in the loop’
- Ethical, model bias and other vital considerations, and more.
In order to focus provide the most value to people in this field—physicians, informaticists, innovation and population health leaders, researchers, and more, we will break out these issues in AI model development and deployment, and make the content available across a series of written blog posts over the coming weeks.
Our panelists for the roundtable include:
- Eran Choman, Chief Clinical Research and Compliance Officer, Medial EarlySign
- Coby Metzger, MD, Data Scientist, Medial EarlySign
- Ben Glicksberg, PhD, Chief Data Science Advisor, Lucem Health
- Andrei Petrus, Senior Manager of AI Solutions, Lucem Health
- Moderator: Marilyn Agbeko, Product, Lucem Health
You can access the recording of the discussion, here.
Setting The Stage
The integration of AI models in lung cancer screening marks a significant advancement in preventive, personalized healthcare, representing a paradigm shift in how we approach early detection and risk assessment for this deadly disease. This innovative approach combines the power of machine learning algorithms with vast amounts of patient data to identify individuals at higher risk of developing lung cancer, potentially months or even years before traditional screening methods would detect any abnormalities.
Why Focus on Lung Cancer?
Lung cancer remains the leading cause of cancer-related deaths globally and in the United States. Recognizing the critical importance of early detection, the US Preventive Services Task Force (USPSTF) has established guidelines for lung cancer screening to identify individuals at higher risk sooner. Initially set in 2013, these guidelines recommended annual screening using low-dose computed tomography (CT) for individuals between 55 and 80 years old with a history of 30 pack-years of smoking, including current smokers or those who have quit within the last 15 years. These criteria underwent significant updates in 2021 to broaden the screening pool. The updated recommendations now include those aged 50 to 80 years with a 20 pack-year smoking history, reflecting an understanding of the earlier onset of lung cancer in some populations. This shift aims to facilitate earlier screening and diagnosis, as lung cancer most commonly presents at advanced stages—stage 3 or 4—severely impacting patients’ quality of life and survival rates.
Despite these expanded criteria, lung cancer screening compliance in the US hovers around single to lower double digits, indicating the majority of the eligible screening population remains unscreened. Current estimates suggest this unscreened demographic represents about 90% of the 50 million individuals eligible under the USPSTF guidelines. This substantial gap in screening compliance presents both a challenge and an opportunity to significantly impact lung cancer detection and outcomes.
Understanding the Data & Feature Selection Process
The foundation of effective AI models for early disease detection lies in the increasing availability and diversity of patient data. Ben Glicksberg emphasizes this point, stating, “The most important thing to realize is the massive growth of available data, especially that data which is being recorded and captured electronically.” He notes the widespread use of electronic health records both nationally and internationally and highlights the integration of various data sources: “digital scans: pathology, x-rays, MRIs are being recorded, captured, stored electronically, and even things like data collected from mobile health apps, and wearables. All these things can represent and capture some aspects of patient state and are being integrated into healthcare analytics.” This comprehensive view of patient health both creates the ability to and enhances an AI model’s potential to detect disease accurately and early. However, the challenge is more than just data collection; it’s also about meticulous selection, preparation and analysis of data from multiple and diverse sources.
Glicksberg elaborates on this issue: “Where features can be identified by experts, like by expert clinicians or clinical practitioners that know of some inherent involvement within the disease diagnosis pathway…on the other side, there is more of like an unsupervised approach where we can take all the data that’s available and utilize methods to see what the models can learn from themselves that are important.” This synergistic process, combining human expertise with machine learning, aims to develop the most effective models for disease detection. By harnessing the wealth of available digital health data and employing this balanced analytical approach, AI models are positioned to make significant strides in early and accurate lung cancer detection, thereby setting the stage to ultimately transform patient outcomes.
Addressing the Challenges with Real-World Data
Real-world data, particularly electronic health records (EHRs), provides a rich tapestry of information on patient health that is invaluable for the development of AI models, including those aimed at detecting lung cancer early. However, as the roundtable experts underscore, this data is fraught with inherent challenges that must be meticulously navigated to ensure the accuracy and reliability of AI tools.
Coding Pollution and Its Implications
At the forefront of these challenges is what Andrei Petrus identifies as “coding pollution,” a term that encapsulates the inaccuracies and discrepancies within EHRs that can lead to incorrect disease identification, amongst many other types of possible identification errors. This issue is primarily due to the operational nature of how diseases are coded in healthcare systems. “Garbage in, garbage out,” Petrus asserts, emphasizing the critical need for high-quality, accurate data as the foundation for reliable AI model construction. Misidentification not only compromises the model’s validity but also its ability to serve its intended purpose effectively.
Building on Petrus’s concerns, Glicksberg delves deeper into the root causes of these data quality issues. He notes the limitations imposed by the primary function of EHRs in operations and billing, which significantly affects how diseases and their subtypes are recorded. “Diagnostic codes…are also not fully meant for research,” Glicksberg points out, highlighting a critical gap between the intended use of these codes and their application in AI-driven health initiatives. The absence of specific codes for newly identified disease forms or subtypes adds another layer of complexity to accurately capturing and categorizing patient conditions.
Navigating Heterogeneity and Bias
Moreover, the practicality of working with real-world data extends to understanding and addressing its heterogeneity and the built-in biases that may affect model development. The unique diagnostic pathways and practices of different health systems introduce a variable that Glicksberg identifies as “specificity bias.” This bias is not a reflection of biological realities but rather the procedural and operational preferences of healthcare institutions. Such biases necessitate a careful, customized approach to model training to ensure the creation of generalizable, reliable AI applications that are not narrowly tailored to the idiosyncrasies of specific datasets or healthcare practices.
The Challenge of Alert Fatigue and the Importance of Specificity
While addressing data heterogeneity and bias is crucial in developing AI models, the challenges don’t end there. The practical deployment of these models in healthcare settings brings its own set of hurdles, particularly in how they interact with and impact healthcare practitioners. One of the most pressing issues in this realm is alert fatigue. Dr. Glicksberg highlights this challenge: “Clinical practitioners are beyond busy. Demands on their time are intense, and they get constant bombardment of alerts that have very low specificity. These alerts actually create a situation they were designed to alleviate. They create an annoyance and a distraction that clinicians are not paying attention to.” The importance of ensuring that alerts are meaningful and specific cannot be overstated, as random or too frequent alerts undermine the very purpose of the AI tool.
And at the same time, as highlighted by Eran Choman, clear communication of AI-driven insights to healthcare professionals is crucial for the effective implementation of these advanced screening tools. “We need to make it as clear as possible… we should make it more than just ‘this individual is at high risk.’… We must create explainability that reveals, in clear language, why a specific individual, based on his or her personal health record, was flagged.” This emphasis on transparency ensures that AI outputs are not only understood but also actionable for clinicians, bridging the gap between complex algorithms and practical medical decision-making in lung cancer prevention.
Tackling the Challenge Head-On
Despite these hurdles, the experts remain optimistic about the potential of real-world data in revolutionizing healthcare through AI. Glicksberg acknowledges the unparalleled value of these data sets, declaring them “absolutely invaluable for the field,” despite the identified challenges. The critical issue then becomes how to effectively clean, standardize, and utilize this data in a way that mitigates the risks of inaccuracies and biases while maximizing its potential to improve patient outcomes through early disease detection and tailored treatment strategies.
What’s Next?
Eran Choman highlights the potential of AI as a transformative solution created with a purpose-built infrastructure to address the challenges inherent in the process. By leveraging AI to enhance screening efficiency and compliance, there’s an opportunity to detect lung cancer at earlier stages when it is more treatable, thereby improving patient outcomes and reducing mortality rates. These insights lay the groundwork for exploring AI-based interventions as a means to support and possibly amplify the effectiveness of low-dose CT screening across the United States, offering an optimistic future for early detection in lung cancer care.
Wrapping Up
Stay tuned for future posts in this series! The expert panel will take these foundations of AI model development and build on them to provide deeper insights into:
- Real-world model validation
- Clinical and financial implications of AI-powered screening
- Setting and measuring benchmarks for success
- Considerations for streamlining the healthcare process and navigating resource constraints