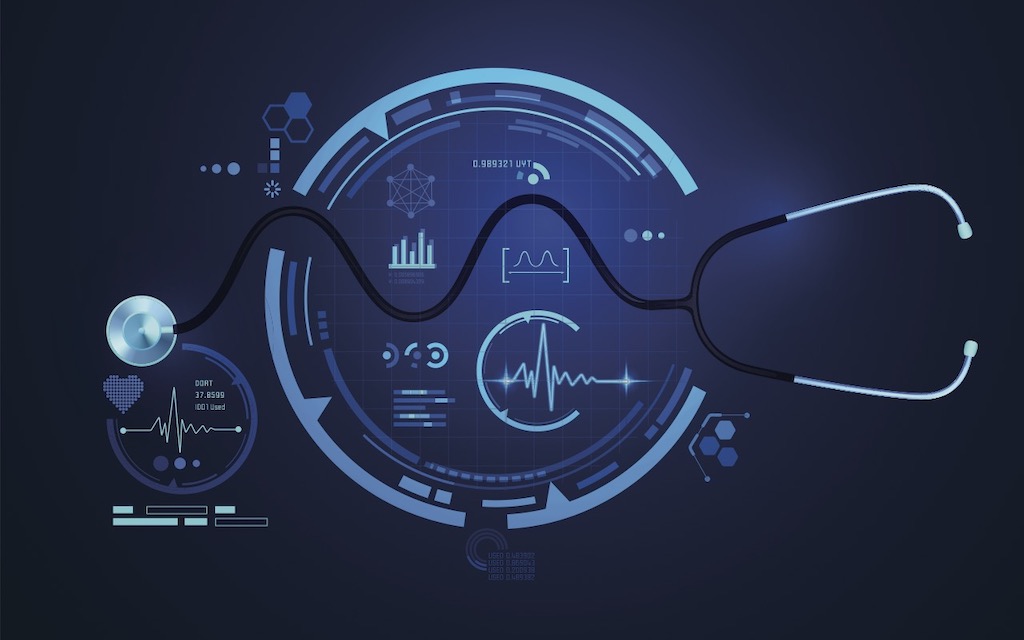
Top Challenges for Clinical Model Deployment: Part 1
AI in Healthcare: Setting a new pace for adoption
Did you know that today, only about half of the world’s businesses report actively using artificial intelligence (AI) in their core business functions[1]? That seems a bit surprising–especially given all the hype and discussion around the potential of AI to transform organizations. But the reality is that many of today’s AI technologies and solutions have yet to demonstrate consistent, practical, and easily scalable value in a business setting. This is especially true in the healthcare industry. So what’s causing this gap between potential and reality for AI in Healthcare? And what will it take to turn AI-powered solutions into trusted and valuable tools that are ready to transform the delivery of healthcare?
1. Collecting, combining, and normalizing all the right data
The foundation of any successful AI solution is quality data (and lots of it). Finding the right data sources for AI models can be challenging in any industry, but it’s especially difficult for healthcare organizations where similar data sets often follow different conventions from system to system.
For example, some pharmaceutical data sources might use the FDA’s National Drug Code (NDC) while others might use a normalized naming system such as RxNorm. To effectively use disparate data conventions in the same AI platform, healthcare organizations need an easy, practical way to recognize and reconcile the differences. In most cases, this involves assembling cross-functional teams that combine the expertise of data scientists, AI model developers, healthcare data specialists, and other IT professionals who collectively understand the nuances of gathering, combining, and normalizing the data that powers clinical AI models.
2. Integrating AI into clinical workflows
Another challenge facing AI in healthcare is the massive gap between the complex mathematical algorithms that power AI models and the frontline clinicians who actually use them. Before AI insights can offer any real value in a clinical setting, they must be connected to a defined action or established workflow. Without these connections, AI will quickly become an irrelevant distraction that frontline healthcare workers simply ignore.
To avoid this unproductive outcome, AI developers need an effective way to translate and input mathematical algorithms into the language of healthcare. AI calculations and predictions need to be delivered through care-focused applications that are thoughtfully integrated with existing clinical tools and technologies. This makes it possible to frame AI insights and predictions in a way that healthcare professionals value and understand.
3. Making quality AI available everywhere
An AI model that works well for one healthcare organization is a good start. But how do you turn a specialized, finely-tuned mathematical model into an effective, scalable solution that consistently performs well across hundreds of different healthcare environments, each with its own unique combination of IT systems, applications, data, and workflows? Individual developers can try to do this themselves, but in most cases, it’s more efficient to partner with experts who already have the skills to successfully deploy, integrate, and scale AI models.
Lucem Health recognizes these challenges and is already working with a cross-disciplinary team of data scientists, software developers, and IT deployment experts to solve them. With an agnostic platform approach, we’re ready to turn the full potential of AI technology as a transformative clinical tool into a practical reality for your organization.
Learn more about how this platform approach works and the challenges it addresses:
[1] https://www.mckinsey.com/business-functions/quantumblack/our-insights/global-survey-the-state-of-ai-in-2020