Five Key Challenges that are Slowing the Progress of AI in Healthcare
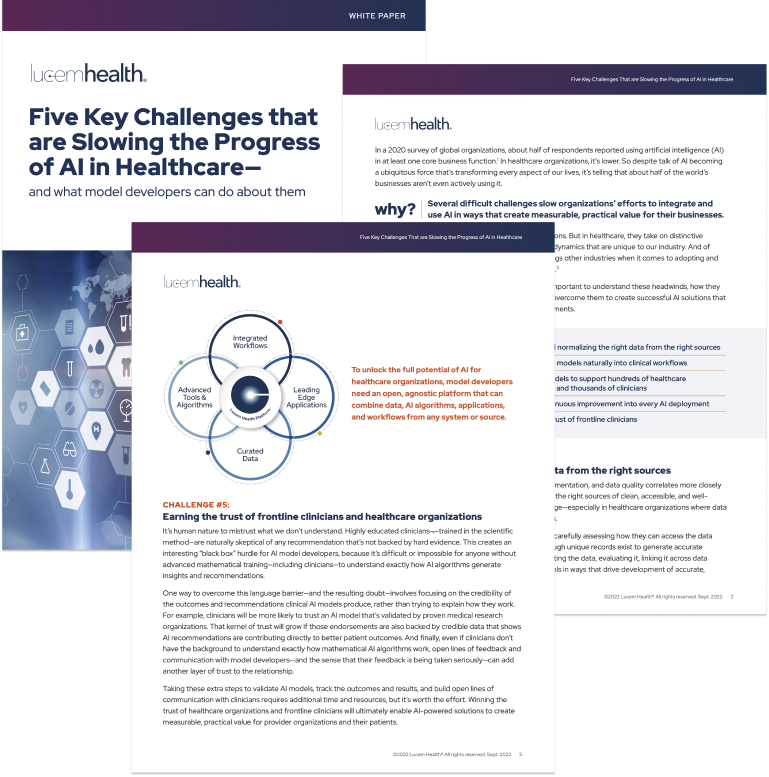
LUCEM HEALTH WHITE PAPER
Bridging the gap between people, process, and AI models
Several difficult challenges slow organizations’ efforts to integrate and use AI in ways that create measurable, practical value for their businesses. These include:
Acquiring and normalizing the right data from the right sources
Integrating AI models naturally into clinical workflows
Scaling AI models to support hundreds of healthcare organizations and thousands of clinicians
Building continuous improvement into every AI deployment
Earning the trust of frontline clinicians
For data scientists in organizations who develop clinical AI models, it’s important to understand these headwinds, how they apply specifically to healthcare, and what it will take to overcome them to create successful AI solutions that improve patient outcomes in real-world clinical environments.