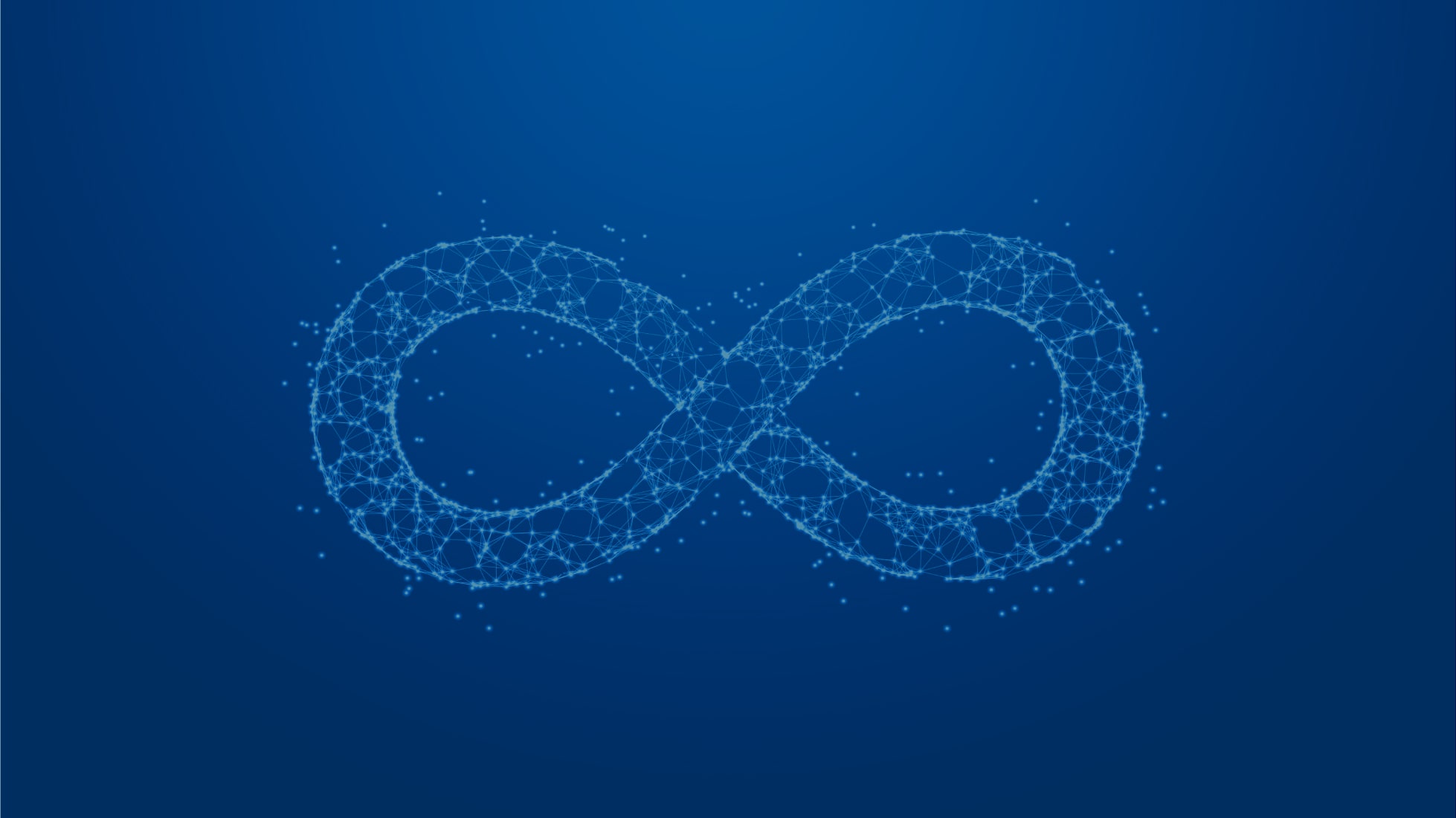
AI Deployment Success: Improving Adaptability Over Time
A recent Becker’s Hospital Review interview with Avishkar Sharma, MD, director of artificial intelligence and a radiologist at Philadelphia-based Einstein Healthcare Network, discusses best practices for successful deployment of AI solutions in healthcare settings.
He notes that many AI tools fail to scale beyond initial pilots because they do not integrate well into clinician workflows. To boost adoption, he recommends involving end users, such as clinicians, early when developing AI solutions to ensure usability in real-world settings. Dr. Sharma emphasizes understanding stakeholder workflows and finding opportunities to enhance them with AI’s capabilities versus replacing human judgment.
Building clinician trust in AI is also critical, so they feel comfortable relying on its recommendations. However, solely focusing on accuracy metrics is not enough – evaluating seamless integration into hospital systems is key. Rather than treating AI deployment as a plug-and-play technology project, he argues that success requires treating it as an iterative, user-centric design process in collaboration with the clinical sites. This human-centered approach allows health systems to maximize the benefits of AI.
At Lucem Health, we strongly agree with the perspectives that Dr. Sharma shares around effective clinical AI deployment. We have found that transitioning AI from proof-of-concept to integral tool lies in co-designing solutions hand-in-hand with frontline providers. We applaud Dr. Sharma for championing these best practices that allow health systems to realize AI’s full potential.
A development process should emphasize understanding clinician workflows first before identifying targeted AI opportunities. It requires regularly collaborating with health systems to evaluate integration factors like usability and interoperability from the start, focusing on building clinician trust in AI through transparency and evidence generation.
Taking Successful AI Deployment A Step Further
Dr. Sharma’s interview does a fantastic job breaking down all of these great points, and we think there is the opportunity for additional commentary that create successful AI deployments – specifically around outcomes data.
As a seasoned healthcare IT executive, specifically in the realm of implementations and customer success, I have seen many technology implementations that are beautifully designed and achieve the desired outcome in the short-term, but the problem lies with adaptability over time. While Dr. Sharma highlights the need for iteration in his interview to an extent, the importance for AI solution insights for continuous improvement are vital for long-term success.
It is commonplace for many machine learning algorithms to adapt and refine with new data. For example, retail and entertainment recommender algorithms often adjust based on new products and user feedback. Such a continuous learning strategy seems apt for clinical AI applications.
A developer could design an AI model to predict the risk of Diabetes within a particular patient group. Continuous learning could be implemented (more on continual learning in this great blog post) to compare these predictions with real patient outcomes gathered from different time periods and diverse sources such as prescribed medications, blood sugar levels, or exercise records. This enables constant model refinement based on its predictions’ alignment with real diagnoses and outcomes. Incorporating broader outcome data equips developers to identify dataset limitations and make ongoing adjustments, improving model accuracy and effectiveness over time.
Though in a typical healthcare setting, various challenges emerge:
- Patient outcome data is stored in multiple isolated systems and organizations.
- Outcome data might not be consistently and properly categorized, with privacy laws limiting patient data usage and sharing.
- Normally, a significant delay of weeks or months hampers the availability of the relevant data for continuous learning from the model’s initial prediction.
Developers must tackle these complex outcome data challenges before continuous improvement of AI can be successful in healthcare.
To address these gaps, more model developers need to be using clinical AI platforms that support comprehensive AI continuous improvement strategies. Effective platforms must support multi-modal, and multi-model capabilities, enabling integration of all data types with any algorithm, and paired with any workflow or application. Hence, it’s possible to collect, merge, and standardize clinical outcome data from diverse sources, aiding developers for easier model enhancement and innovation.
Putting it all together
While Dr. Sharma provides invaluable insights on effective clinical AI deployment from an organizational change management perspective, realizing the full potential of these tools also requires a focus on continuous improvement fueled by outcomes data. Developers must implement continuous learning strategies that enable models to be refined over time based on new patient diagnoses, treatment responses, health indicators from diverse sources, and feedback from end users at provider organizations. This allows for ongoing enhancements to model accuracy, clinical effectiveness, and adoption. However, harnessing the necessary outcome data poses challenges around integration, standardization, privacy, and timeliness.
Clinical AI platforms that can consolidate multi-modal data across systems, while ensuring proper data governance, are key to empowering model developers with the elements needed for robust and responsive AI advancement. With both diligent human-centered design and continuous technical refinement through comprehensive data, it becomes possible to deliver AI that evolves in step with clinical needs and realities. This fusion promises to unlock AI’s immense possibilities for enhancing medical expertise and patient care.
If you’d like to learn more about successful AI deployment and continuous improvement, check out our white paper, or get in touch with our expert team.