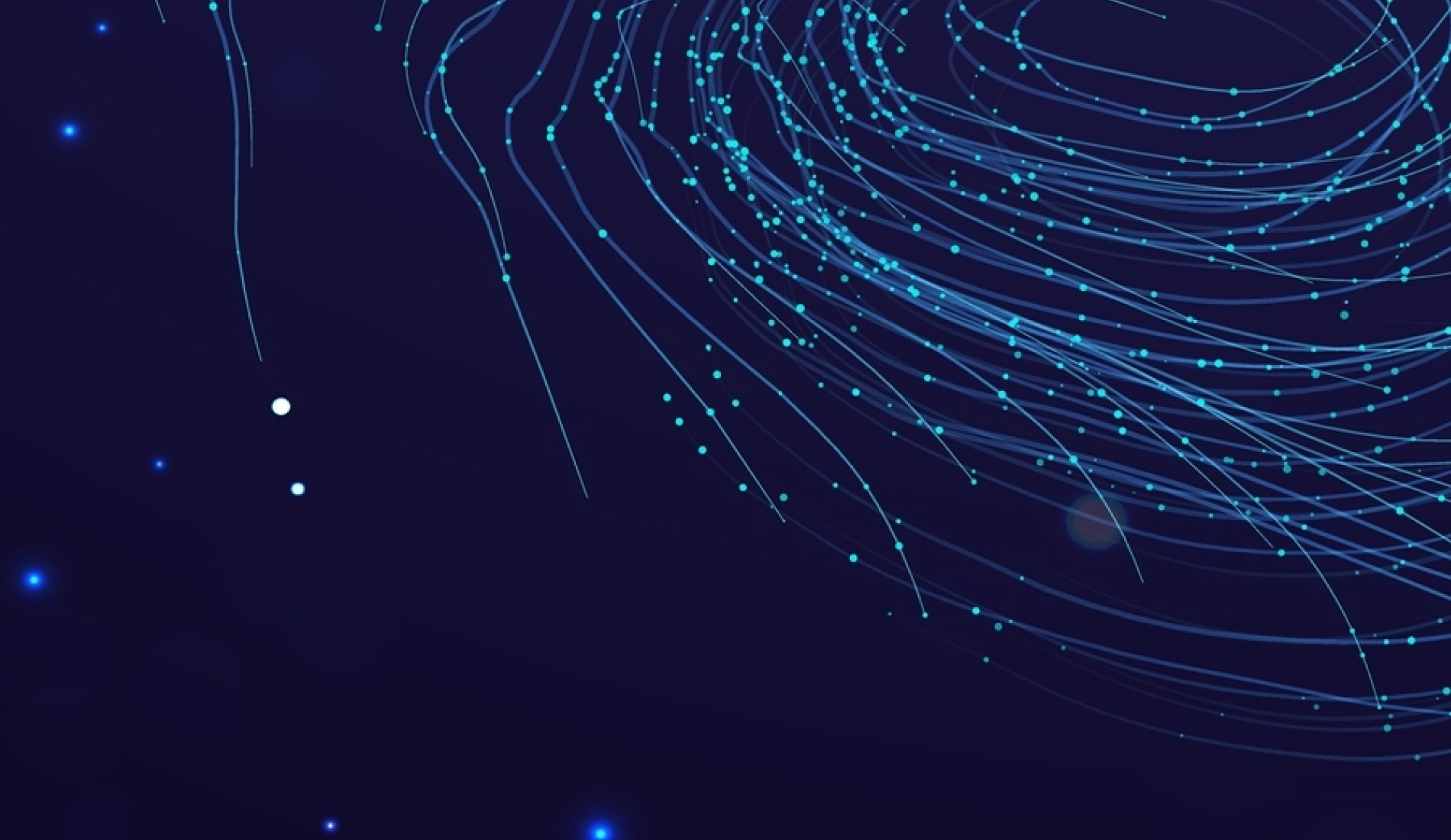
Hiding in Plain Sight: Leveraging Existing Patient Data for Early Detection of Diabetes
Introduction
Sophisticated artificial intelligence (AI) algorithms capable of mining electronic health record (EHR) data can reveal patients at a higher risk for undiagnosed diabetes. This proactive, early risk identification through advanced analytics can lead to targeted preventive interventions that may help avoid the complications of uncontrolled diabetes down the line.
The Alarming Scale of the Diabetes Epidemic
The prevalence of diabetes has reached epidemic proportions in the United States. According to authoritative estimates from the Centers for Disease Control and Prevention (CDC), over 37 million Americans currently have diabetes, with another 1.5 million new cases diagnosed every year. [1] If current trends continue, as many as 1 in 3 American adults could have diabetes by 2050. [2]
It is estimated that nearly 25% of diabetes cases nationwide remain undiagnosed. [3] This means millions of Americans have diabetes but are unaware of their condition and are not receiving essential treatment to prevent complications. Furthermore, with specialists like endocrinologists only seeing a small fraction of the total patient population, many individuals with early diabetes warning signs slip through the cracks of the healthcare system, leading to countless preventable adverse outcomes.
Uncontrolled diabetes can lead to an array of serious complications including kidney failure, blindness, heart disease, stroke, and lower limb amputation. The staggering costs of treating diabetes and associated complications have skyrocketed to an estimated $327 billion in 2017 when both direct medical expenditures and lost productivity are included. This represents a more than threefold increase since just 2007. [4]
Telltale Risk Signals Are Already Hiding in Patient Data
Detailed clinical data from electronic health records, insurance claims records, prescription drug history, lab test results, and other sources may contain important early indicators and risk predictors that can be leveraged to uncover patients with undiagnosed diabetes before complications occur. The key is utilizing advanced clinical machine learning to extract these vital risk insights from complex but readily available datasets.
Digital health company Medial EarlySign realized that by applying sophisticated machine learning algorithms to routine patient data already being collected by healthcare providers, they could identify specific individuals likely to be diagnosed with diabetes months or years before they are aware that they actually have the disease. Among individuals without known diabetes and without up-to-date blood glucose and HbA1c results, the model accurately identifies those who are at high risk for having diabetes today. Their predictive model is based on historical EHR data including demographics, vital signs, diagnoses codes, medications, and lab results. It also requires no active patient participation.
“The value of early detection is crucial because it allows for timely intervention and management. Swift diagnosis enables individuals to adopt healthier lifestyles, receive proper medical guidance, and prevent serious complications such as heart disease, kidney damage, and nerve problems,” commented Or Geva, EarlySign co-founder and CEO. “Effective early intervention and personalized care can significantly improve the quality of life and reduce the long-term health risks associated with diabetes. Our partnership with Lucem help to achieve the aims of providing higher quality care while lessoning societal impact.”
The Multifold Benefits of Early Risk Detection for Diabetes
AI models for early detection of those at risk of currently having diabetes can yield numerous important benefits:
- Patients experience improved long-term health outcomes through earlier use of preventive lifestyle modification programs and metformin drug therapy after being screened following identification of high diabetes risk. [5]
- Health systems and insurers can reduce spending on extremely costly hospitalizations and medications to treat devastating downstream diabetes complications including kidney failure, heart attacks, blindness, and lower limb amputation.
- Primary care physicians, specialists like endocrinologists, and broader clinical care teams can optimize use of limited resources and time by focusing screening and preventive efforts on the highest-risk subset of the patient population.
Continuing Our Hypothetical Example on Impact of Early Diabetes Detection
Let’s continue the hypothetical example from our last post, and imagine a regional health system that deployed Reveal for Diabetes to detect undiagnosed diabetes among its patient population. In this health system population of 50,000, using Reveal for Diabetes that targets the 3% of the highest risk patients for diabetes would generate 209 likely undiagnosed diabetics from 1,500 screenings. If you were to attempt to find those 209 undiagnosed diabetics via traditional screening methods, you would have to screen nearly 7,500 patients. That is 5 times the resources required compared to using Reveal for Diabetes for early diabetes detection.
By using Reveal for Diabetes tied to the health system’s diabetes care management program or population health initiatives, they would see a $749,000 financial benefit over three years. (This assumes only 50% of revealed patients agree to screening).
Both clinically and financially, AI-powered solutions make a significant case for the future of diabetes detection.
The Bright Future on the Horizon for Data-Driven Diabetes Care
As real-world evidence accumulates, AI models could be deployed at scale across entire populations to vastly expand early screening and detection of diabetes risk. Paired with continually improving models and solutions, healthcare systems can finally shift towards the proactive, preventive care paradigm that is needed to bend the diabetes cost curve and dramatically improve outcomes.
Geva continued, “Combining the platform expertise and care management background of Lucem Health with proven and validated models from EarlySign creates a powerful solution for health systems seeking to contain the spiraling cost of care while also promoting community health initiatives for vulnerable populations.”
Sophisticated AI modeling of electronic health record data can unlock subtle but powerful diabetes risk predictors hiding in plain sight. By empowering earlier detection, complications can be reduced or avoided through timely preventive interventions. Thus, AI-driven diabetes detection represents a foundational pillar of the preventive care model needed to help control this growing epidemic.
Note: This is part of a larger blog series for early disease detection developed in collaboration with our model developer partner, Medial EarlySign. Stay tuned for our final blog post next week that dives into early detection of colorectal cancer! You can find all the previous posts, here: