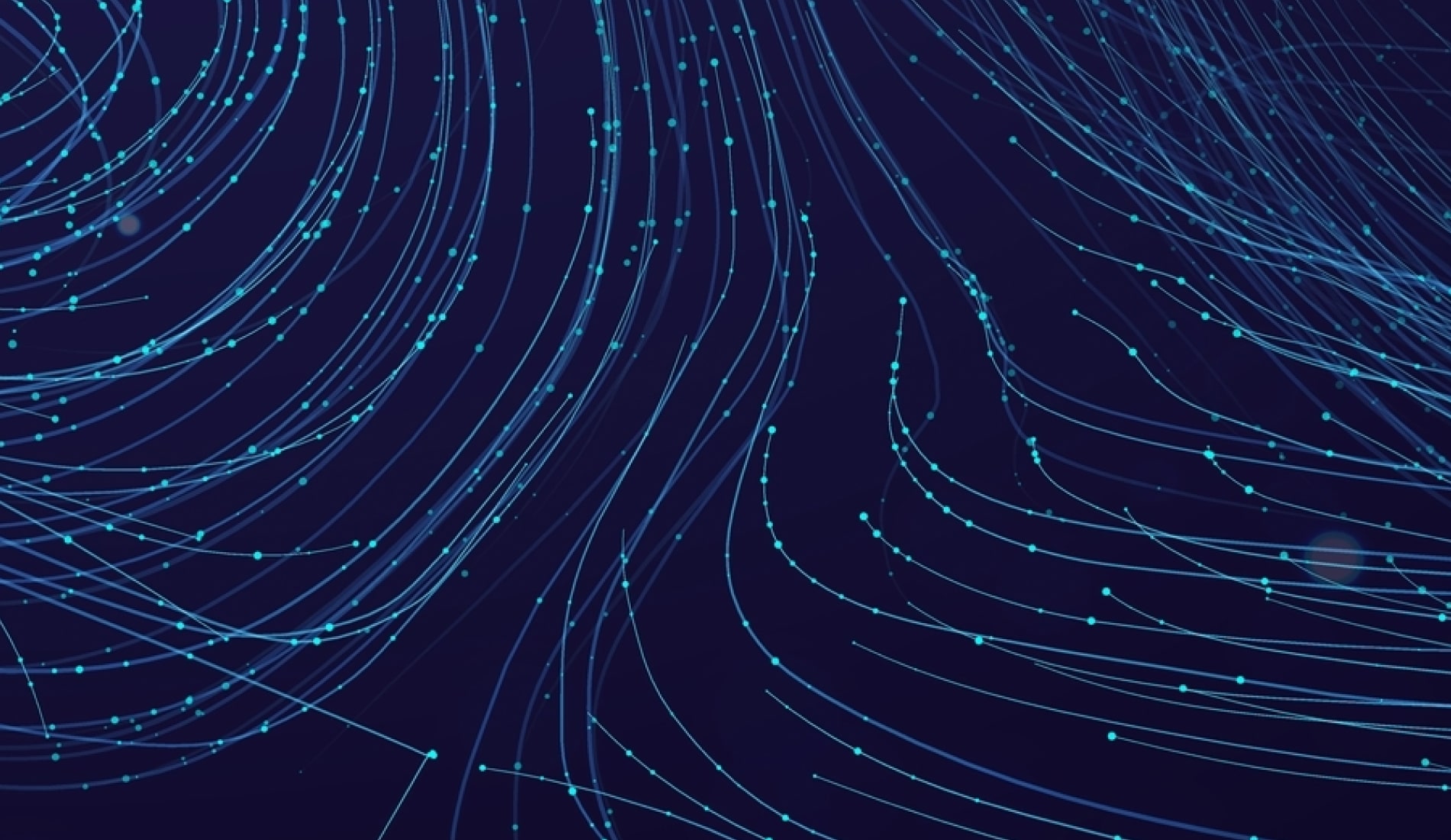
Colorectal Cancer Screening at Scale: AI-Driven Population Health
Introduction
Colorectal cancer (CRC) poses a major public health burden as the third most commonly diagnosed cancer and second leading cause of cancer deaths in the United States. In 2022 alone, an estimated 106,180 new cases of colon cancer and 44,850 new cases of rectal cancer will be diagnosed, while an estimated 52,580 people will die from colorectal cancer this year. [1] Although incidence and mortality rates have slowly declined over the past few decades due to increased awareness and screening, colorectal cancer remains a highly prevalent cancer. Yet CRC could be the most preventable—and successfully treatable–if caught in its early stages. Identification of those at highest risk of harboring colorectal cancer and early detection through screening is critical, as colorectal cancer is often asymptomatic when it is most treatable: in its early stages.
Colorectal Cancer Incidence and Mortality
According to American Cancer Society statistics, colorectal cancer incidence rates decreased by 2.6% per year among those 50 and older from 2008 to 2017. Meanwhile, death rates dropped by 3.0% per year from 2008 to 2019 in this same age group. However, worrisome trends are emerging among younger adults. For those under age 50, incidence rates increased by 2% per year from 2012 to 2016. [2] Looking ahead, the National Cancer Institute predicts the number of new colorectal cancer cases per year in the United States will rise from 147,950 in 2020 to 183,240 by 2030, reflecting the aging and growth of the population. [3]
Survival Advantage from Early Detection
When detected at an early, localized stage before spreading, the 5-year survival rate for colorectal cancer is 90%. However, only 14% of patients with distant metastatic disease survive 5 years post-diagnosis. [4] This stark contrast underscores the vital need for early detection, as symptoms of colorectal cancer often do not appear until the disease has advanced. Approximately 22% of colorectal cancer patients have disease classified as late-stage at diagnosis, indicating the cancer has already spread. [4] Screening to detect and remove precancerous polyps or diagnose cancer at an early stage provides the best opportunity for survival and even prevention through polyp removal before malignancy occurs.
Current Suboptimal Screening Rates
Regular screening is imperative for early colorectal cancer detection, yet rates remain below targets. In 2020, approximately 69.4% of adults ages 50-75 were up-to-date with USPSTF colorectal cancer screening guidelines, falling short of the 80% goal. [5] With recent updates to screening guidelines including the expansion of screening for those ages 45-49, it will be imperative to keep up with opportunities for early detection.
Screening compliance varies by factors like insurance status, race, education level, and access barriers. Strategies are needed to increase screening uptake through a combination of people, technology, and process: public education campaigns, improved affordability and access, provider interventions like reminders and outreach, broadening the range of screening options with emerging technologies like stool DNA tests, and last but not least, advanced AI/ML algorithms. Boosting screening rates will allow more precancerous polyps to be prevented from progressing and enable more cancers to be caught at early stages when highly treatable – saving more lives.
A Practical AI Model for Detecting Colorectal Cancer Risk
Seeing an opportunity to improve early detection of CRC, Medial EarlySign developed an intelligent machine learning algorithm, LGI-Flag, that may identify those at higher risk of Lower Gastro-Intestinal (LGI) complications—including CRC—that could be used to highlight those who are overdue and merit extra encouragement for CRC screening. The algorithm uses existing patient EHR data: age, sex, and CBC results (while excluding those with hemoglobin gene defects).
“Early attempts by AI technology vendors to conquer the healthcare sector were often guided by over-ambition, seeking overly large amounts of data, and falling short of the expectations created,” commented Ori Geva, EarlySign co-founder and CEO. “We created a lean model, using simple and available data so it would be easy to deploy and could support multiple implementation use cases in manageable projects. We thoroughly validated the model in multiple external datasets and deployed it with leading partners like Geisinger and demonstrated consistent performance and value retrospectively and prospectively.”
When deployed at Geisinger as part of a care management solution and studied over a 63-week period across 25,610 patients, the LGI-Flag model found that of those flagged at higher risk who scheduled a colonoscopy (68%), 70% of the patients had a significant finding [6]:
- 51% were detected with an Adenoma or Polyp
- 8% were found to have CRC at some stage
- 11% had some other Lower GI finding
More Real-World Results
Across three additional studies at Maccabi Health System in Israel, Kaiser Permanente in Northern California, and an analysis using 2.5 million patient records from Practice Research Datalink data from the UK, the model proved effective in CRC detection, along with other lower GI disorders.
- Maccabi Health System conducted the first study on approximately 80,000 individuals in Israel, using the LGI-Flag tool to identify 688 people as high-risk for colon cancer. Following colonoscopies, 7.5% of these individuals were diagnosed with CRC. [7]
- In the second study utilizing data from Kaiser Permanente Northern California, high accuracy was reported, especially in early detection, with CRC diagnosis within the next six months marking an odds ratio (OR) of 13.1 in early-stage CRC and 24.8 in advanced-stage CRC for patients with high scores. LGI-Flag was capable of predicting both proximal and distal cancers. [8]
- The third study on UK patient data involved a large sample of over 2.5 million patients aged 40 years and older. The LGI-Flag model demonstrated 77.6% accuracy for predicting colon cancer diagnosis within an 18-24 month period. It was able to demonstrate a positive predictive value (PPV) of 8.8% of correctly identify the individuals who were later diagnosed with colon cancer among the flagged population. [9]
Scaling AI-Driven Colorectal Cancer Screening Efforts
With a proven solution studied across multiple different population sets on different continents, Medial EarlySign looked for opportunities to expand deployment of the LGI-Flag model in an effective, efficient manner. Understanding the nearly endless list of considerations when deploying new technology into health systems, EarlySign quickly saw the opportunity of partnering with Lucem Health. In swift order, the partners were able to package the LGI-Flag model as part of a larger AI-driven platform—specifically a lower GI detection solution, Reveal for Lower GI, that seamlessly integrates with health system existing IT resources and clinical workflows.
The Growing Opportunity for Population-Level Colorectal Cancer Screening
AI-driven risk stratification transforms the approach to colorectal cancer prevention and treatment from a single patient perspective to a population health one. While individual care is crucial, an emphasis on overall population health allows for broad-scale improvements in health outcomes. By proactively identifying patterns and potential risks among large groups of individuals, AI contributes to comprehensive disease prevention strategies and structural healthcare changes that benefit the broader population.
This approach also may reduce health disparities across different communities. High-value patient interactions, driven by AI, ensure that every individual within a population receives care that aligns with their unique needs, ultimately elevating the overall health status of the community. In turn, this strengthens the strained capacity of healthcare systems to respond effectively to health needs.
Moreover, AI’s capability of improving resource allocation is essential in population health management. Resources targeted towards identified high-risk groups can lead to the prevention of disease on a large scale, enhancing the health of the entire population. By utilizing AI in predicting to mitigate the downstream effects of colorectal cancer, we can transition from a reactive healthcare model to a proactive, population health-focused one. This results in healthier communities, efficient healthcare systems, and a significant decrease in human suffering caused by this disease.
Ready to reveal hidden CRC and lower GI risks efficiently and at-scale, all without further burdening provider resources and major disruptions to your existing clinical operations? Talk to our team today! Or check out our recent white paper on early disease detection.
Note: This is part of a larger blog series for early disease detection developed in collaboration with our model developer partner, Medial EarlySign. Stay tuned for our final blog post next week that dives into early detection of colorectal cancer! You can find all the previous posts, here:
- Prevention is Key: How AI-driven Early Detection Can Enhance Population Health Outcomes
- From Data to Decision: How AI Streamlines Early Identification of those at Highest Risk of Progression from Prediabetes to Type 2 Diabetes
- Hiding in Plain Sight: Leveraging Existing Patient Data for Early Detection of Diabetes